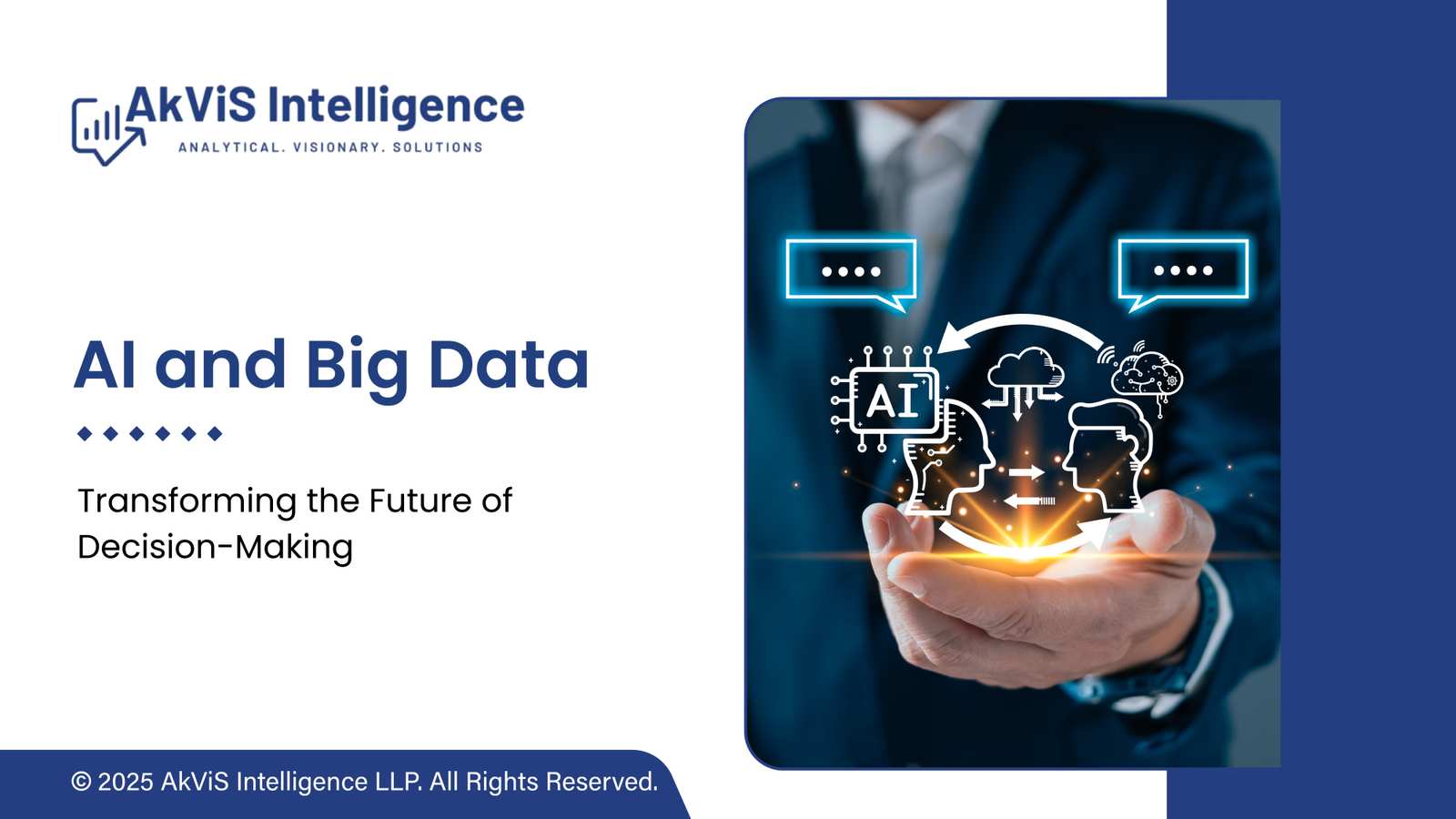
What is Artificial Intelligence?
Artificial Intelligence (AI) refers to the simulation of human intelligence in machines created to reason, learn, and make choices. AI systems are designed to perform tasks that typically require human intelligence, such as problem-solving, making decisions, understanding language, perceiving information, and adapting to new data.
What is Big Data?
Big Data refers to enormous and complex data collections that conventional data processing techniques and tools find challenging to handle proficiently. It typically includes vast quantities of data generated from various sources, such as social media, sensors, transactions, and devices, and may be structured, semi-structured, or unstructured.
How Big Data and AI Work Together
- Data Collection: Big Data encompasses the gathering of extensive amounts of structured and unstructured data from various sources, including sensors, social media sites, customer interactions, and other channels. This data acts as the foundation for AI applications.
- Data Storage and Processing: Technologies for large-scale data such as Hadoop and Spark facilitate the storage and handling of vast datasets. This system ensures that the data is accessible and usable by AI algorithms.
- Data Preparation: Before AI can analyze the data, it generally requires some preparation. This stage entails cleaning, transforming, and structuring the data to ready it for machine learning models.
- AI Modeling: The cleaned data is then analyzed using machine learning algorithms, which fall under the umbrella of AI. These algorithms could include supervised learning for predictions, unsupervised learning for detecting patterns, and reinforcement learning for decision-making.
- Training and Inference: AI models rely on past data to recognize patterns and relationships. Once trained, they can swiftly produce predictions or decisions based on incoming data.
- Insight Generation: The final outcome of this procedure is actionable insights. AI algorithms reveal hidden patterns, anomalies, trends, and predictions from Big Data, which can fulfill various roles, from improving products and services to optimizing business operations.
What is the Best AI for Big Data?
- Machine Learning: Machine learning serves as a core element of AI for Big Data. It encompasses different methods such as supervised learning, unsupervised learning, and deep learning. For example, supervised learning is applied in classification and regression tasks, making it ideal for predictive analytics involving Big Data.
- Natural Language Processing (NLP): NLP is a branch of AI that emphasizes the communication between computers and human language. It is especially useful for examining unstructured text data, like customer reviews, social media updates, or news pieces, on a larger scale.
- Computer Vision: Computer vision allows machines to analyze and comprehend visual data from the environment, such as images and videos. This technology is essential for activities such as image recognition, object detection, and facial recognition, which can be utilized in Big Data situations.
- Reinforcement Learning: Reinforcement learning algorithms can be utilized in situations where making decisions is vital. They are ideally suited for enhancing intricate systems and processes, like supply chain management or self-driving cars, by learning via interaction.
- Deep Learning: A portion of machine learning, deep learning utilizes neural networks that comprise numerous layers. It is particularly useful for activities that demand great precision in pattern identification, like speech recognition or image categorization.
Examples & Applications of AI for Big Data
Big data and AI play essential roles in data analysis and predictive analytics, utilizing technology to simplify and speed up the process. This reduces the time from data collection to insight, can lessen errors in the procedure, and may result in cost savings.
AI's influence on Big Data has been substantial across multiple sectors:
- Healthcare: AI is employed to evaluate patient information, aid in identifying illnesses, forecast patient results, and even tailor treatment strategies based on unique health records.
- Finance: Financial organizations employ AI for identifying fraud, algorithmic trading, evaluating credit risk, and customer service chatbots.
- Retail: AI-based recommendation systems tailor shopping experiences, enhance inventory control, and offer flexible pricing strategies.
- Manufacturing: AI-driven predictive maintenance minimizes downtime by anticipating equipment breakdowns, whereas quality control systems improve product quality.
- Marketing: AI improves marketing initiatives by examining customer behavior, categorizing audiences, and refining advertisement targeting.
Artificial Intelligence and Big Data: Similarities and Differences
The combination of Artificial Intelligence and Big Data creates a strong synergy that allows organizations to extract insights from their vast and complex datasets. Utilizing the capabilities of AI-driven algorithms, businesses can streamline data evaluation, gain predictive insights, and uncover hidden patterns that enable informed decision-making.
While AI and Big Data are distinct areas, they share similarities and differences:
Similarities:
- Data-Centric: Both AI and Big Data rely on data for their fundamental operation. AI requires large datasets for training, and Big Data supplies these datasets.
- Machine Learning: AI relies heavily on machine learning, which is an element of both areas. Machine learning algorithms are created with Big Data to produce forecasts and decisions.
Alternatives:
- Scope: Big Data concentrates on collecting, storing, and managing extensive data volumes, while AI aims at creating algorithms and models for tasks like pattern recognition and decision-making.
- Objective: The primary goal of Big Data is to structure and analyze information, while AI focuses on creating intelligent systems that can perform tasks on their own.
Possible Upcoming Trends in AI and Analytics:
- Progress in Data Management and Governance: The data lakehouse, a groundbreaking idea that combines the versatility of data lakes with the strong management features of data warehouses, is increasingly essential in a time of substantial data expansion. This method addresses the varied requirements of contemporary data analytics, offering scalable storage and effective data processing capacities essential for AI-based insights and decision-making.
- Focus on Data Privacy and Security: As advanced technologies such as generative AI emerge, there is a growing emphasis on enhancing data privacy and security protocols. Organizations are moving towards enhanced data security methods, understanding the significance of safeguarding sensitive data from breaches and maintaining compliance with changing data protection laws.
- Expanding Use of AI and Machine Learning: Innovations like NLP and AutoML are changing how we interact with data, simplifying complex information and making it easier to understand. NLP connects human language with digital information. Simultaneously, AutoML streamlines the application of machine learning models to practical issues, enhancing accessibility to AI for those without expertise.
- Enhancing Operational Efficiency via Automation: Financial pressures are pushing companies to adopt automation in data analysis. Technologies such as data automation, cloud analytics, and decision intelligence are optimizing processes, allowing businesses to manage extensive data quantities more efficiently and to make quicker data-driven choices.
- Improved Accessibility and Democratization of Data: The movement towards data democratization focuses on ensuring that data is reachable and comprehensible to a wider range of individuals within organizations. This entails creating tools and platforms that allow non-technical users to interact with data analytics, promoting a more inclusive data culture.
- Data Quality and Governance: There is an increasing focus on guaranteeing the quality and governance of data. This includes putting into action frameworks and practices that ensure data accuracy, consistency, and security. Proper data governance is crucial for organizations to gain precise insights and uphold confidence in their data analytics efforts.
- Emerging and Innovative Technologies: Investigating generative AI and Quantum Computing is creating new possibilities in data analysis. Generative AI facilitates the development of novel, synthetic data types, whereas Quantum Computing is set to transform data processing through its exceptional speed and efficiency.
- Social and Ethical Factors: With the increasing integration of AI and data analytics in business functions, there is a growing emphasis on their social and ethical ramifications. This means making sure that these technologies are applied in an ethical, transparent manner that aligns with societal values and standards.
- Challenges and Opportunities in Analytics Maturity: The Alteryx Analytics Maturity Assessment reveals persistent difficulties in maximizing the capabilities of data analytics and AI. Numerous organizations continue to struggle with effectively utilizing the potential of data analytics and AI. This encompasses obstacles related to incorporating advanced analytics into business operations, enhancing employee skills, and fostering a culture that promotes data-driven decision-making.
Conclusion:
Artificial Intelligence (AI) and Big Data have revolutionized how organizations process, analyze, and utilize data, driving innovation and efficiency across multiple industries. AI simulates human intelligence, enabling machines to perform tasks such as decision-making, problem-solving, and language comprehension. Big Data, on the other hand, focuses on managing vast and complex datasets generated from diverse sources like social media, sensors, and transactions. Together, they form a powerful synergy that transforms raw data into actionable insights.
AI relies on Big Data for training and refining its algorithms, while Big Data uses AI to analyze patterns, predict outcomes, and automate decision-making. This partnership is evident in applications ranging from personalized healthcare to fraud detection in finance, and from predictive maintenance in manufacturing to targeted marketing strategies.
Future trends highlight the increasing focus on data privacy, innovative technologies like Quantum Computing and generative AI, and democratizing data for broader accessibility. However, challenges remain in governance, analytics maturity, and ethical considerations. As industries continue to embrace these advancements, AI and Big Data will play a pivotal role in enabling smarter, faster, and more ethical
Related Article
"Wind Energy: Powering a Sustainable Future"
18-02-2025